Midpoint Rule¶
In the midpoint rule you approximate the area under the curve as a rectangle with the height as the function value at the midpoint of the interval:
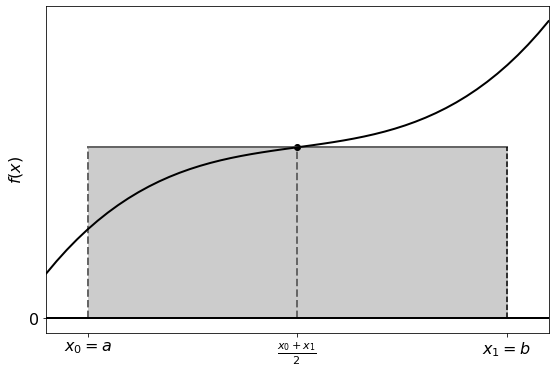
Composite Midpoint Rule¶
For a more accurate solution we can subdivide the interval further, constructing rectangles for each subinterval, with the function value of the midpoint used as the height:
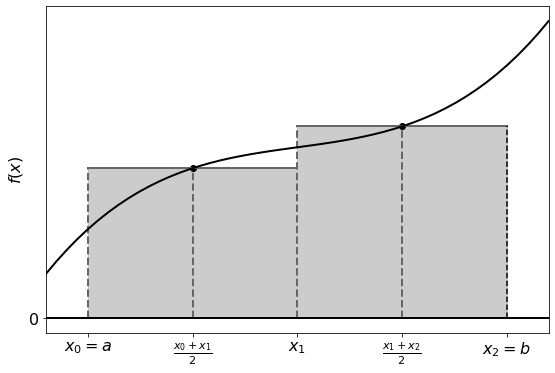
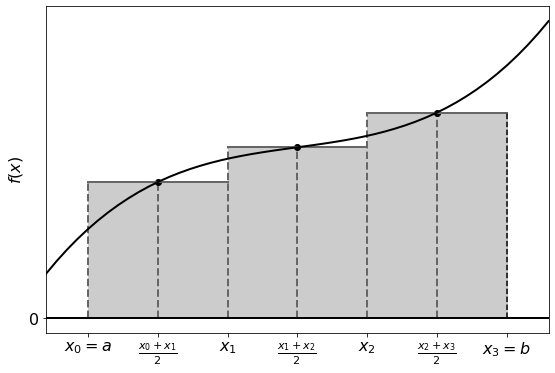
For \(n\) subdivisions:
If these divisions are equal, then
which makes the approximation:
Assuming that \(n\) is chosen so that \(0 < \tfrac{b - a}{n} < 1\), the error for this method is \(O\left(\tfrac{1}{n}^3\right)\) [IntMid1].
Composite Midpoint Rule with a Discrete Data Set¶
Let’s consider the case where we have a discrete set of data points \((x_i, y_i)\) for \(i = 0, \dots, n\), where:
We want to approximate the integral of \(f(x)\) using this data and the midpoint rule. We can treat each \(x_i\) as the midpoint (except for \(x_0\) and \(x_n\) at the boundaries) and determine the size of the interval around it using the adjacent values.
For equally spaced data points, where \(\Delta x = x_i - x_{i-1}\) is constant, we can approximate the integral as:
where the first and final contributions are halved as the intervals they represent are halved (note that these aren’t at the midpoints of their intervals, rather at the boundaries).
References¶
- IntMid1
James F. Epperson. An Introduction to Numerical Methods and Analysis. John Wiley & Sons, Inc., Hoboken, New Jersey, second edition edition, 2013.